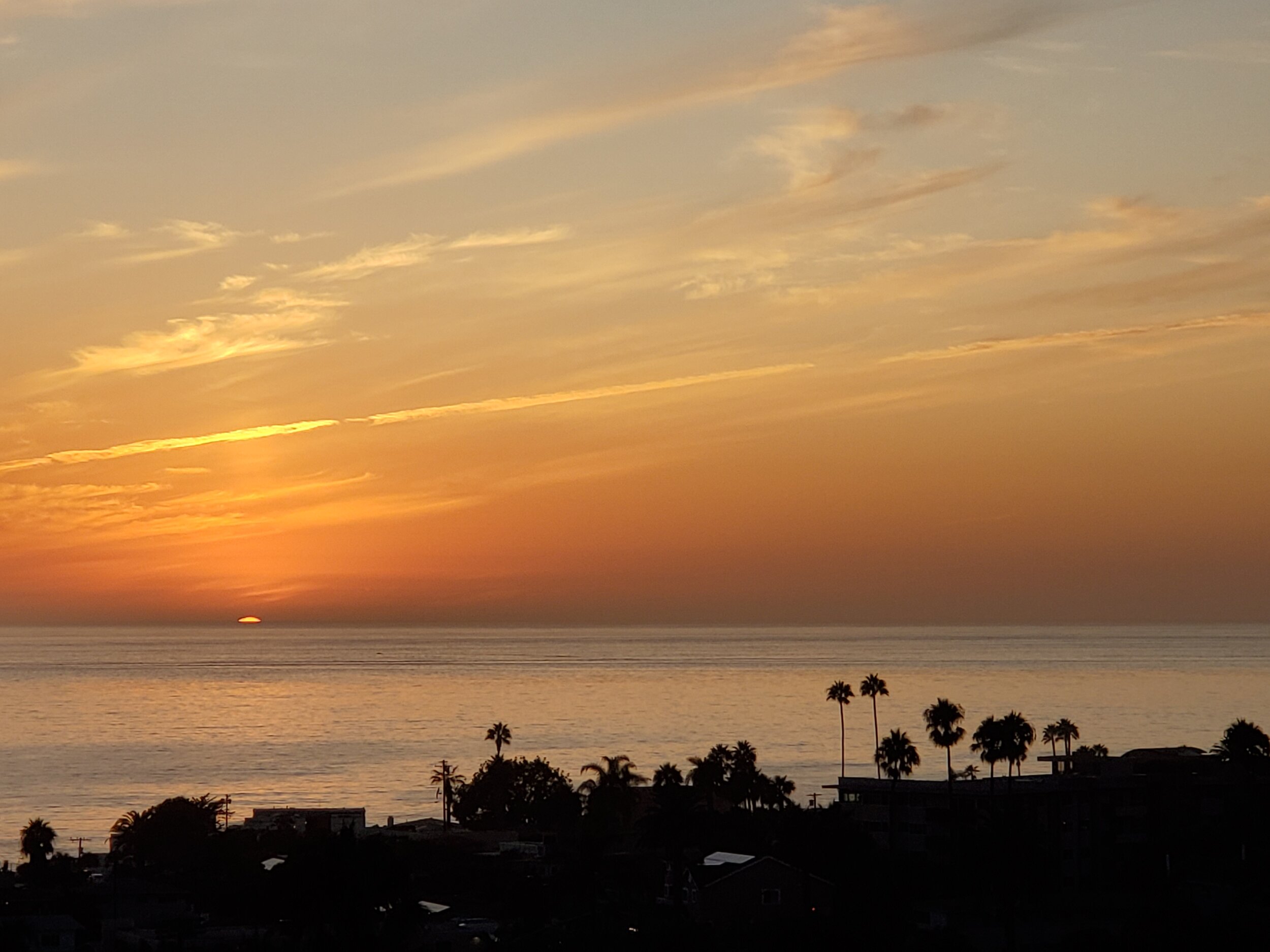
AI & Data Science Tech Blog
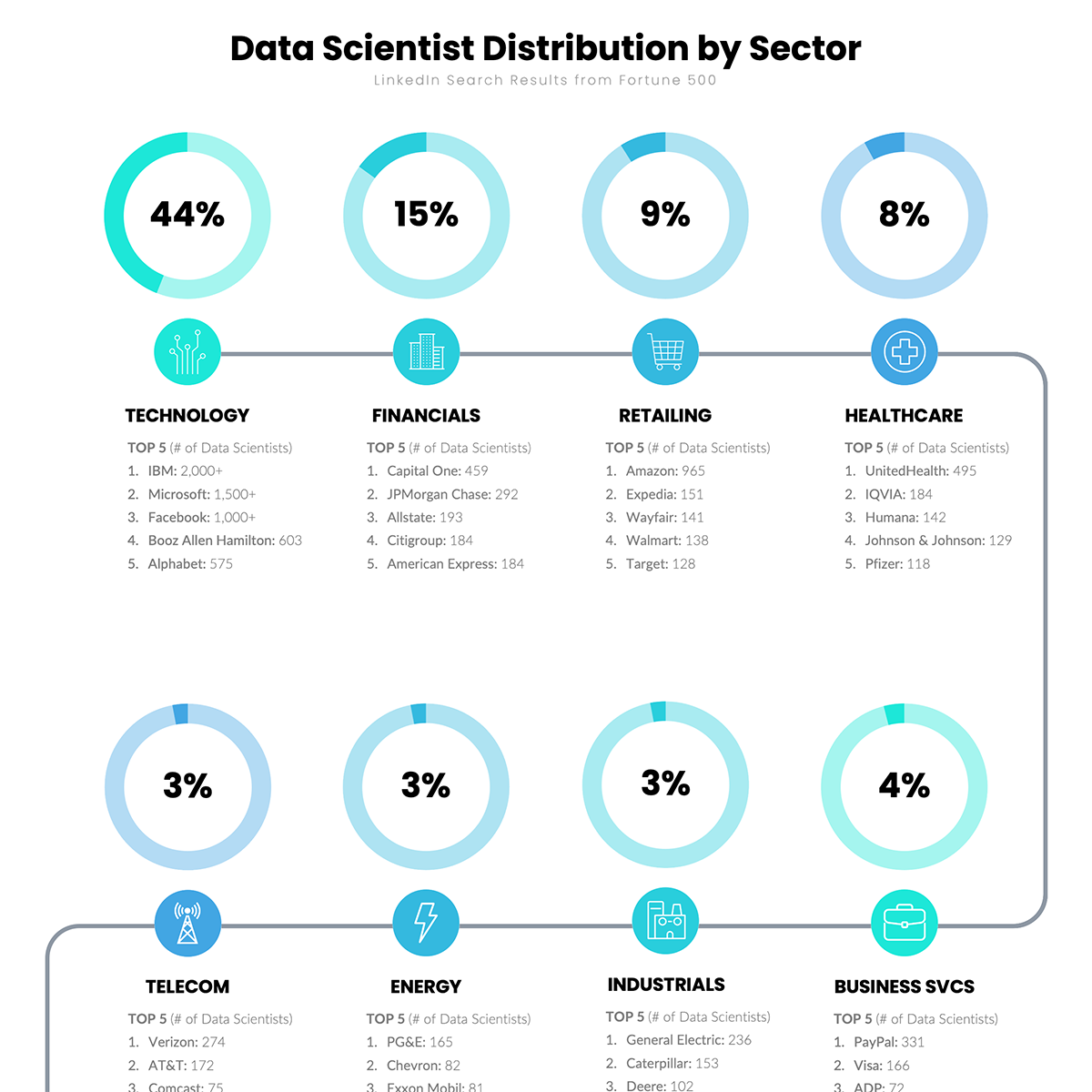
Ranked: Infographic of the Fortune 500 with the Most Data Scientists
Infographic Ranking: Businesses & Sectors of Fortune 500 with the Most Data Science & Machine Learning Projects
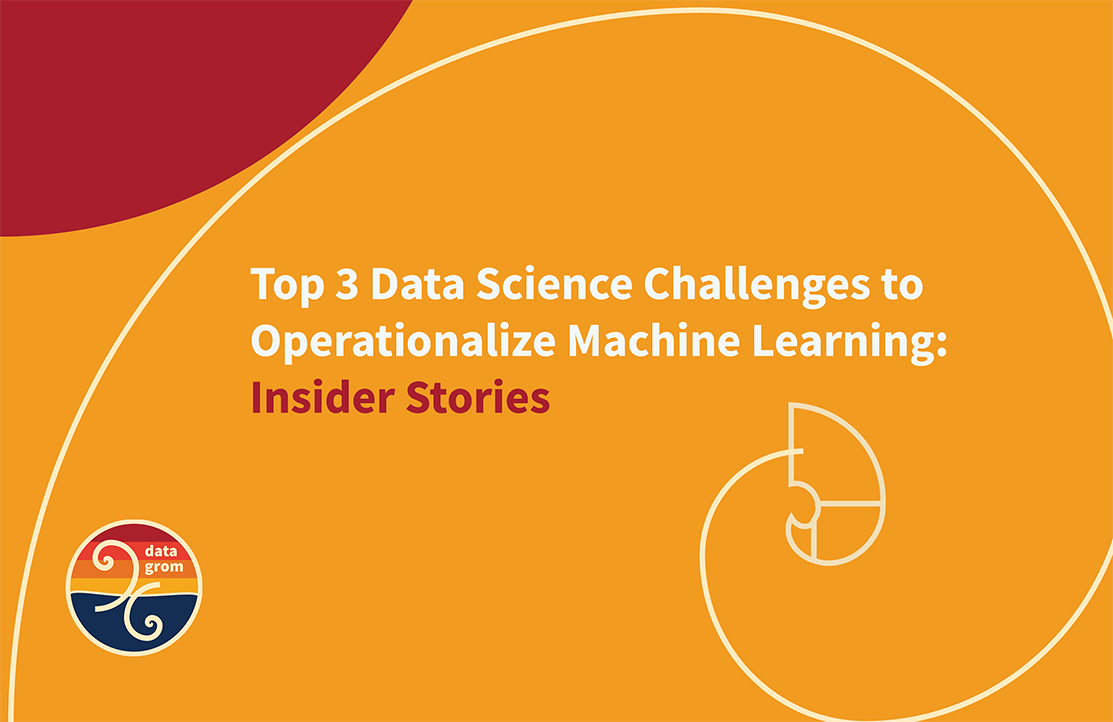
Top 3 Data Science Challenges to Operationalize Machine Learning: Insider Stories
Want to add data science and machine learning capabilities to your arsenal (or just make more data-driven decisions)? If so, I commend you! While the rewards can be plentiful, quite often so are the roadblocks to achieving this goal. To help with your journey, I wrote this article to give you a preview of the top challenges you will likely face, so that you can immediately start to make adjustments to your business in order to facilitate this effort.
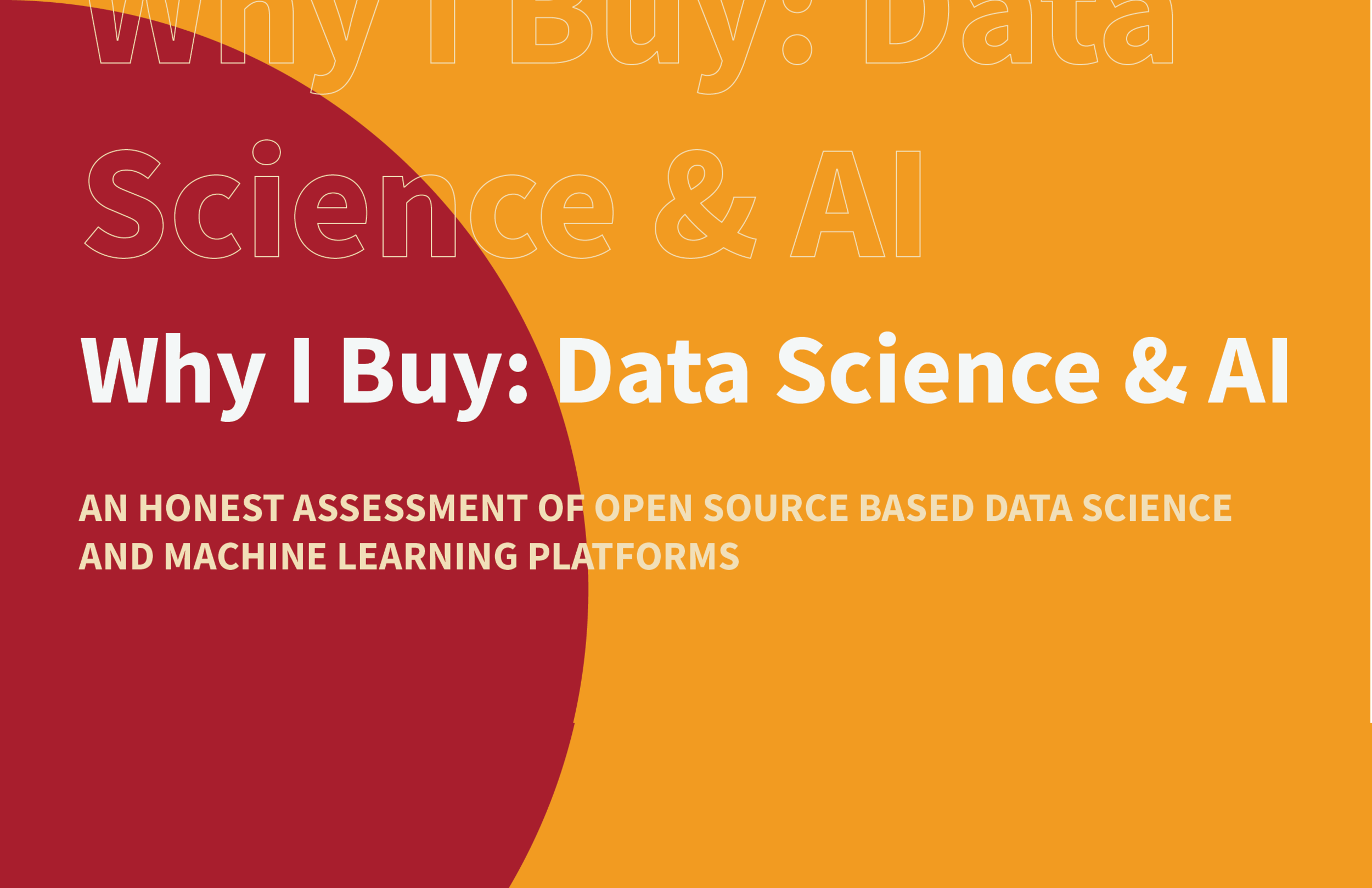
My Take on the Gartner MQ for Data Science and Machine Learning Platforms: “Why I buy” Data Science and Machine Learning Platform Edition
Update: Gartner has postponed its Data and Analytics Conference. Hopefully, this article helps to inform in that conference’s absence. If you have any questions about my comments, please comment below, or send me a note.
A few weeks ago, Gartner released its 2020 Machine Learning and Data Science platform Magic Quadrant. Each year Gartner uses their “Completeness of Vision /Ability to Execute” methodology to categorize and rank each vendor who participates in the annual Gartner dance. The Gartner report claims to provide clarity on a complicated market. I believe it creates total confusion. (Yes, this I know that’s their model)
As a veteran of the machine learning and data science technology market, I have some of my own thoughts on these products, their value proposition, their targeted personas and their actual personas reached. I have paid close attention to the MQ for the last 5 years and have worked closely with and for many of the companies/technologies in the quadrant. I wanted to share some of my thoughts in a series of posts. This is the first.